As the AI landscape evolves, integrating AI-based technologies into knowledge management becomes crucial for businesses aiming to stay ahead. Welcome to our blog series, "Revolutionizing Knowledge with AI: A Guide to RAG and Semantic Search”, where we delve into how Retrieval-Augmented Generation (RAG) and semantic search can transform technical knowledge, making it more accessible, accurate, and contextually relevant for your users.
This blog series will consist of three posts, each building on the previous one, to gradually unfold the complexities and benefits of using RAG and semantic search in AI applications for technical content:
- Elevating AI Responses for Technical Content: The Power of Retrieval-Augmented Generation
- Transforming Technical Content Search: The Benefits and Mechanics of Semantic Search for RAG
- GraphRAG: Future Directions in AI and Documentation
Elevating AI Responses for Technical Content: The Power of Retrieval-Augmented Generation
In this post, we explore how Retrieval-Augmented Generation (RAG) can elevate AI responses, making knowledge more accessible, accurate, and contextually relevant. Discover how integrating advanced AI technologies into knowledge management is essential for businesses striving to stay ahead in the rapidly evolving landscape of AI.
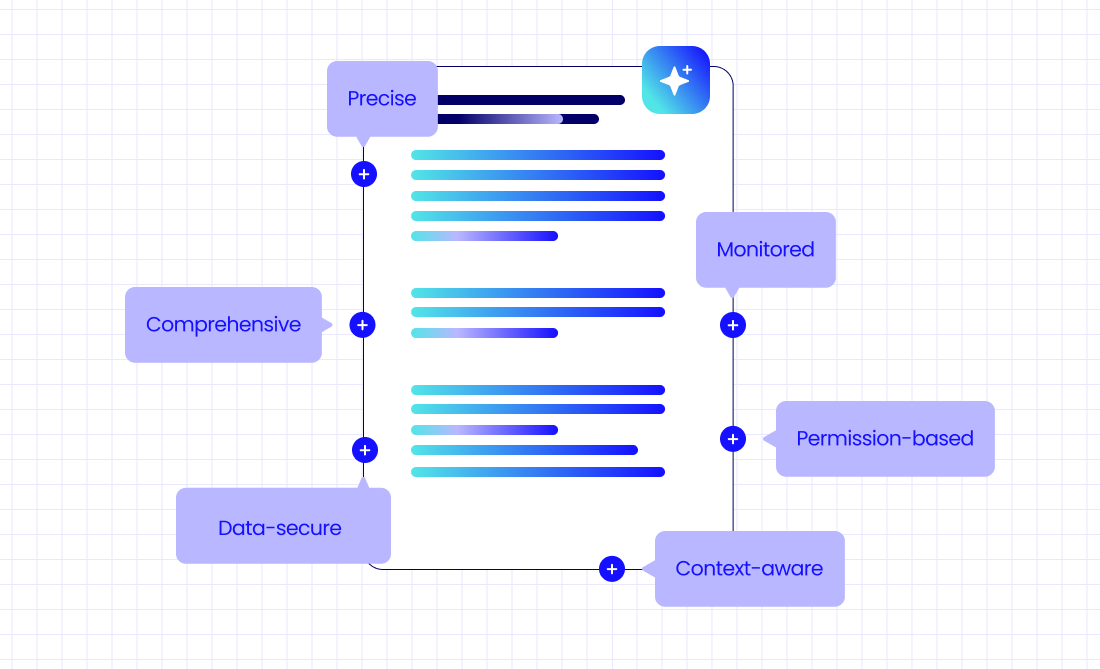
AI for Technical Content – Why It’s Important and Challenging
Leveraging AI for technical content is essential for delivering accurate, contextually relevant answers to users, providing them with the modern, streamlined experience they now expect. However, while users are familiar with AI-based engines that generate responses from general, non-specialized content, when it comes to product-specific documentation, AI-generated answers need to be:
- Comprehensive: enterprise knowledge is scattered across various silos due to the use of multiple authoring tools and repositories, such as Confluence, Google Drive, SharePoint, and others. For the AI-generated answer to be as comprehensive as possible, it has to have all the relevant information from these content silos.
- Precise: Users use documentation to perform tasks with products, and expect it to be highly accurate. Therefore, the AI-generated response to their question must be precise, rely solely on the official technical content, and with no hallucinations.
- Context-aware: You may have technical content about various products in multiple versions. But when a user asks a generic question, they expect the generated answer to be specific to their use case. For instance, if they ask “How to install product A?”, they expect the answer to be applicable to the version they are using, otherwise it may be misleading.
- Permission-based: Users have different content viewing permission levels, which ensures they can only view what they are entitled to. This is why the AI-generated response must only rely on content the user is entitled to see.
- Data-secure: In the world of AI and large language models (LLMs), ensuring data security is paramount. It is crucial that the LLM used to generate AI responses does not save confidential data or utilize it for further training. This practice safeguards sensitive information, maintaining user privacy and trust, while ensuring compliance with data protection regulations.
- Monitored: It's crucial to continuously monitor AI-generated responses to ensure they remain accurate and relevant over time. Regularly auditing the AI's output helps identify potential issues, such as outdated information or subtle inaccuracies that could mislead users. This ongoing oversight is essential for maintaining the quality and reliability of AI-generated knowledge.
How Zoomin Prepares Customers to Integrate AI Solutions
For years, Zoomin has been the leading provider of knowledge governance solutions. This experience has led us to develop a platform that is tailored specifically for structured and unstructured knowledge, setting us apart in the industry. Here's how:
Coverage – We Integrate Your Siloed Content
For years, we at Zoomin have been developing a wide variety of content connectors to pull in data from various sources, and continue to do so even today. Once the data is ingested from the different sources into the Zoomin Platform, it is normalized to a consistent format to ensure that all information adheres to a uniform structure, facilitating easier processing and utilization by AI systems.
Precision – No Hallucinations, Answers Are Provided Only Based on the Actual Content
One of the key differentiators of our platform is the elimination of hallucinations. By grounding the AI-generated responses in the organization's knowledge, we ensure that they are accurate and reliable. This approach not only enhances user trust but also improves the overall user experience. Hallucinations in AI-generated content can lead to misinformation and erode user confidence.
Context Awareness – Provide Relevant Answers
In collaboration with our customers, we process content uploaded to the platform to accurately label it based on the organization's unique taxonomy, such as by product and version. This labeling capability allows the AI to refine responses based on exact context, providing users with accurate and tailored information that directly addresses their unique needs and situations.
Permissioning – We Protect Sensitive Content
Our permission model is the cornerstone of our platform, ensuring that sensitive content is protected and only accessible to authorized users. This model not only safeguards proprietary information but also enhances the accuracy and relevance of the responses generated by our AI applications. By implementing granular access controls, we ensure that users get access to information that is appropriate for their role and permissions within the organization.
Data Security – Your Content Is Your Content
At Zoomin, we prioritize the security and integrity of your data. We prevent third-party vendors from using your content to train their models, ensuring your intellectual property remains protected. Additionally, we employ advanced encryption techniques to safeguard your data both at rest and in transit, maintaining the confidentiality and security of your information at all times.
Analytics – Actionable Insights into AI Interactions
Zoomin's platform is integrated with an advanced analytics system that provides a comprehensive view of user questions, responses provided by Zoomin’s AI applications, and user feedback. The data provided by Zoomin Analytics allows organizations to identify user behavior trends, evaluate the accuracy and relevance of the AI-generated answers, and assess the coverage of the applications. These insights help our customers continuously optimize their AI applications, to deliver accurate and relevant information to their users.
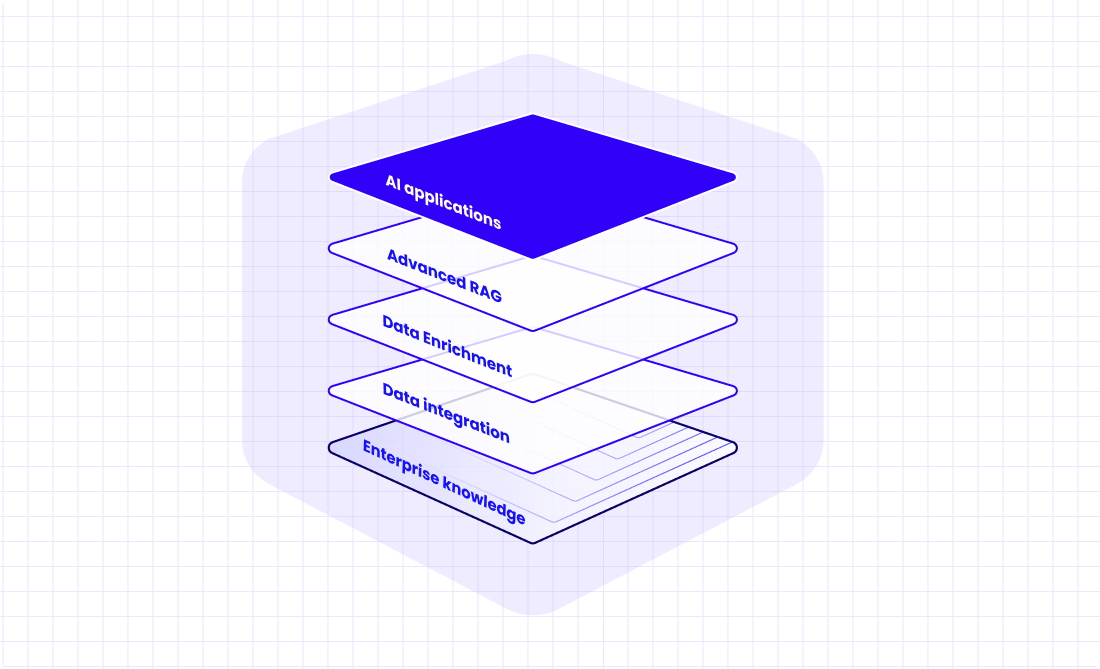
Enter RAG
RAG (Retrieval-Augmented Generation) is an innovative approach that combines retrieval-based methods, which pull relevant information from a predefined set of documents, with generation-based models, which craft responses based on that information. This combination produces more accurate and contextually relevant answers. By integrating these two methods, RAG addresses some of the limitations of traditional AI models, particularly when handling complex queries and generating precise responses.
How Does RAG Work?
The RAG process begins with a user query, which is first processed by a retrieval engine to identify relevant documents or snippets. These retrieved pieces of information are then used to construct a prompt for the generation model, which produces a response based on the augmented prompt. This two-step process ensures that the generated content is both contextually relevant and accurate.
The retrieval engine plays a crucial role in the RAG process: using advanced search algorithms to find the best matches, it is able to determine which documents or snippets are most relevant to the user's query. Once the relevant documents are retrieved, they are used to create a prompt that provides the necessary context for the generation model.
Based on this augmented prompt, the generation model (LLM) then produces a response. This response is grounded in the factual information retrieved by the retrieval engine, ensuring that it is both accurate and relevant. This combination of retrieval and generation methods provides a powerful tool for answering complex queries and generating precise responses.
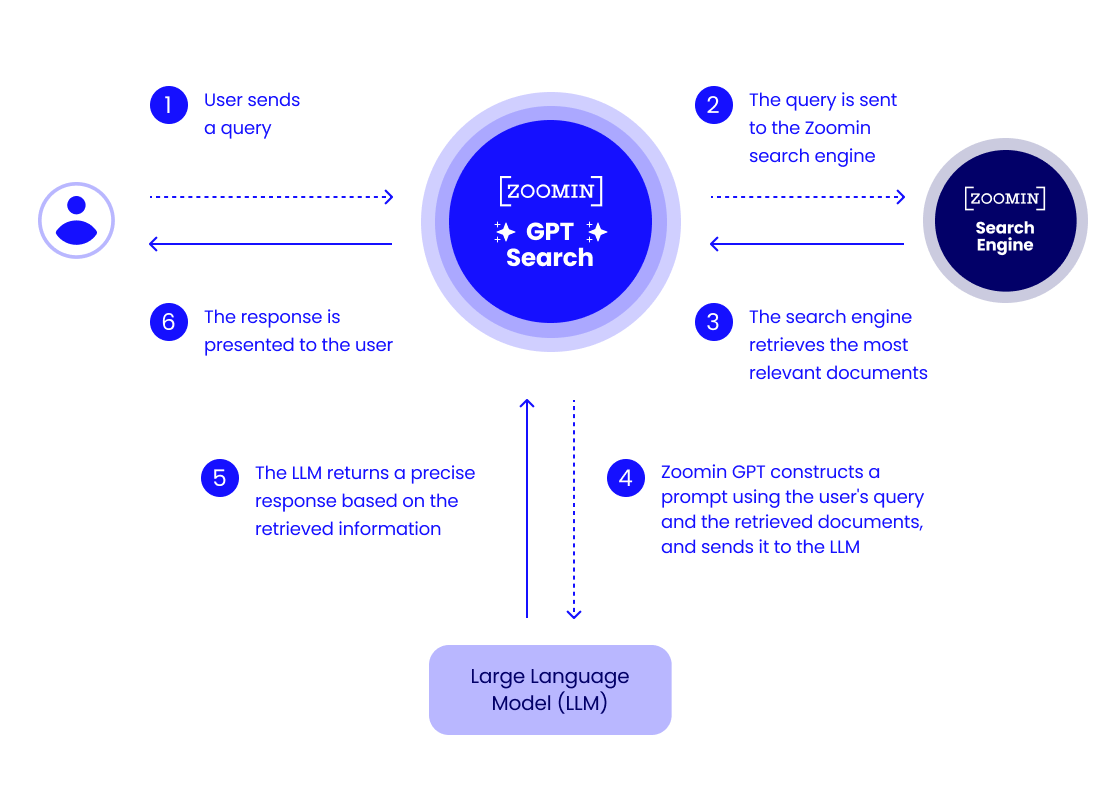
How Zoomin Leverages RAG in Its AI Applications
At Zoomin, we leverage RAG in our AI applications to deliver highly accurate and contextually relevant responses. For example, when a user queries our Zoomin GPT Search, the question is processed by our advanced search engine, which retrieves the most relevant documents. These documents are then used to craft a prompt for the LLM, which generates a precise response based on the retrieved information. This approach not only enhances the accuracy of the responses but also ensures that they are grounded in the organization's knowledge base.
In our Zoomin Conversational GPT application, the RAG process enables more dynamic and context-aware interactions. Users can engage in natural language conversations with the AI tool, receiving responses that are both informative and relevant. This capability is particularly valuable for technical support and customer service, where accurate and timely information is critical.
By leveraging RAG, we can provide users with a more engaging and effective experience. The ability to retrieve and generate content that is both accurate and contextually relevant sets our AI applications apart, delivering significant value to our customers and their users.
How You Can Leverage Zoomin for Your AI Applications
Zoomin provides customers with a seamless AI integration experience. Our platform supports both headless content solutions, where customers connect their AI applications, and a comprehensive solution that includes our AI applications. This flexibility allows customers to choose the level of integration that best suits their needs.
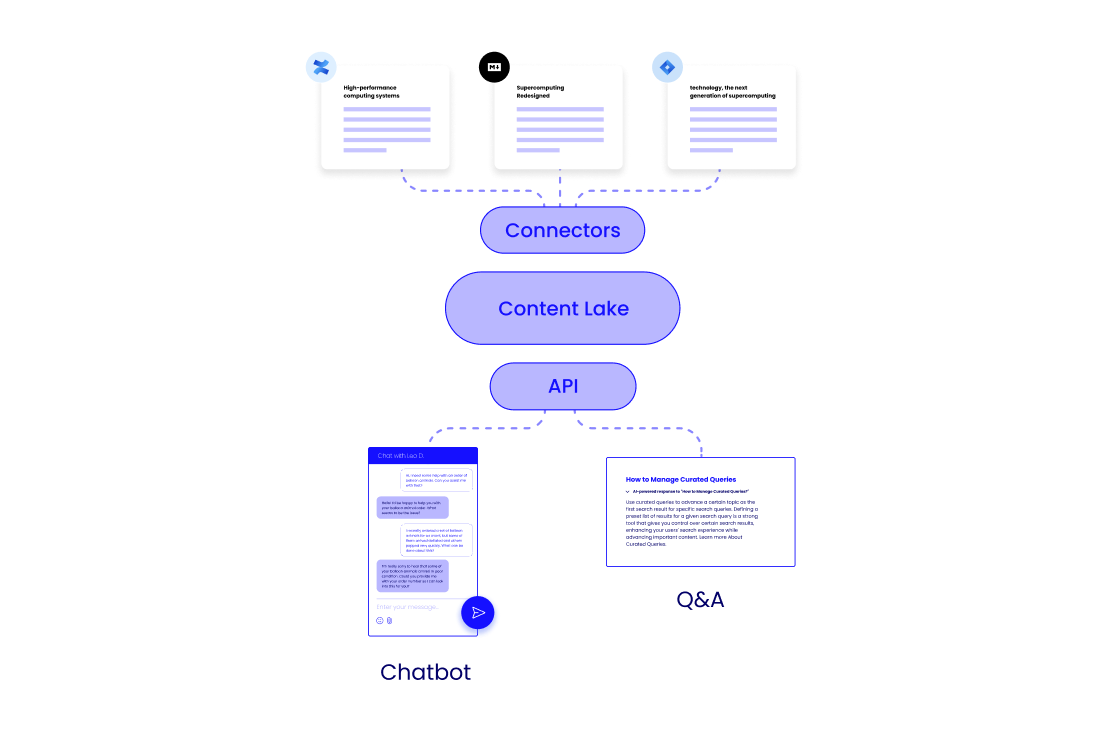
Platform Only – Build Your Own AI Applications
Customers can utilize our platform to facilitate advanced RAG (or even train) their own AI models, leveraging our robust content ingestion and management capabilities. This approach allows for a highly customized AI integration that aligns with their specific needs. In addition, customers can use our platform to develop RAG-based AI integrations to provide their users with the most accurate, comprehensive AI-generated response that is based on their technical content.
Platform + Applications – A Comprehensive AI Solution for Technical Content
Alternatively, customers can opt for our comprehensive solution, which includes our AI applications such as Zoomin GPT Search and Zoomin Conversational GPT, which already utilize RAG to deliver precise, context-aware responses, enhancing the overall user experience. Our AI applications are designed to be intuitive and user-friendly, allowing customers to leverage advanced AI capabilities to get the information they need. These applications are continually updated and improved, ensuring that customers always have access to the latest advancements in AI technology.
What’s Next?
We discussed Zoomin’s solution for integrating AI into technical content management and its unique approach to handling the challenges associated with documentation. By leveraging Retrieval-Augmented Generation (RAG), Zoomin ensures that AI-generated responses are precise, context-aware, and based solely on verified content, minimizing the risk of misinformation. Stay tuned for our next post, where we will explore the benefits and mechanics of Semantic Search for RAG, delving deeper into how this technology enhances the AI experience in documentation.